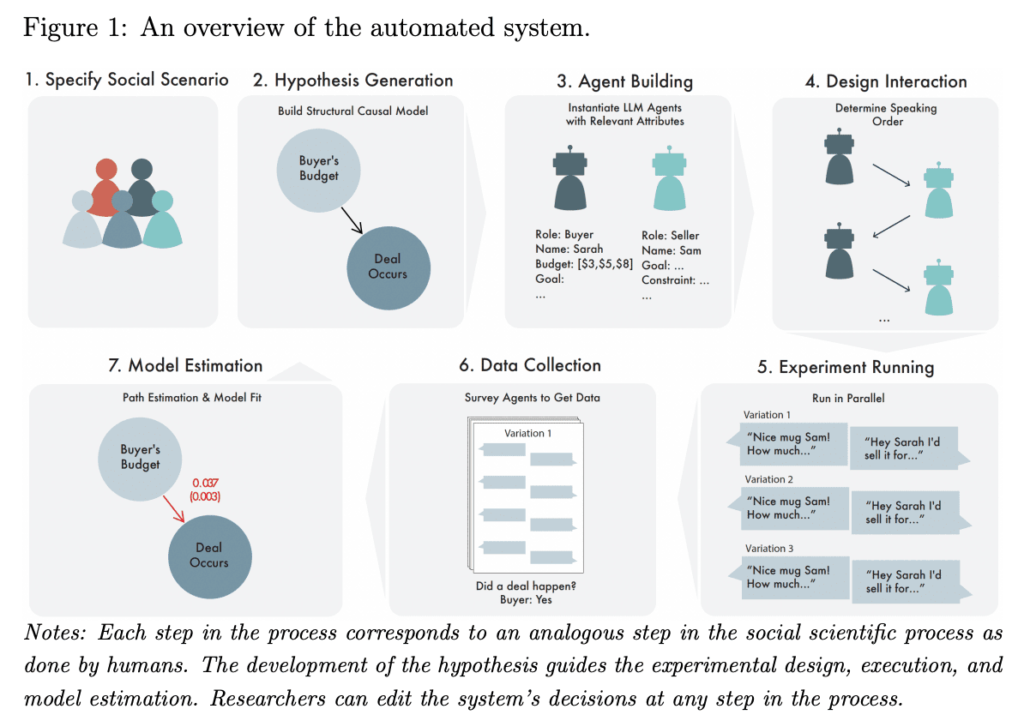
Revolutionizing Social Science Research with AI
In a groundbreaking collaboration, researchers from MIT and Harvard are pushing the boundaries of social science with the introduction of a sophisticated AI-powered system. This new technology leverages large language models (LLMs) and structural causal models to automate the process of developing and testing theories across various social scenarios. This initiative marks a significant step forward in the integration of artificial intelligence into empirical social science research.
Introduction to the AI-Driven Social Science Research System
The collaboration between these esteemed institutions has produced a system that combines advanced computational models with deep learning to explore and understand complex social behaviours. By using structural causal models—mathematical tools that define cause-and-effect relationships—the system can create detailed agents and scenarios that mimic real-world interactions.
The Role of Structural Causal Models in Experiment Design
What are Structural Causal Models?
Structural causal models are crucial in this research as they provide a framework for understanding how variables influence each other in a controlled setting. These models guide the AI system in generating realistic and dynamic agents that behave in ways that reflect genuine human interactions, thus allowing for a seamless transition from theoretical exploration to practical experimentation.
Testing the System: Social Scenarios from Bail Hearings to Auctions
Researchers tested the AI system across a variety of settings, including bargaining sessions, bail hearings, job interviews, and auctions. In these experiments, the system not only generated agents and scenarios but also proposed and tested hypotheses autonomously. This demonstrated the system’s capability to navigate and analyze complex social interactions effectively.
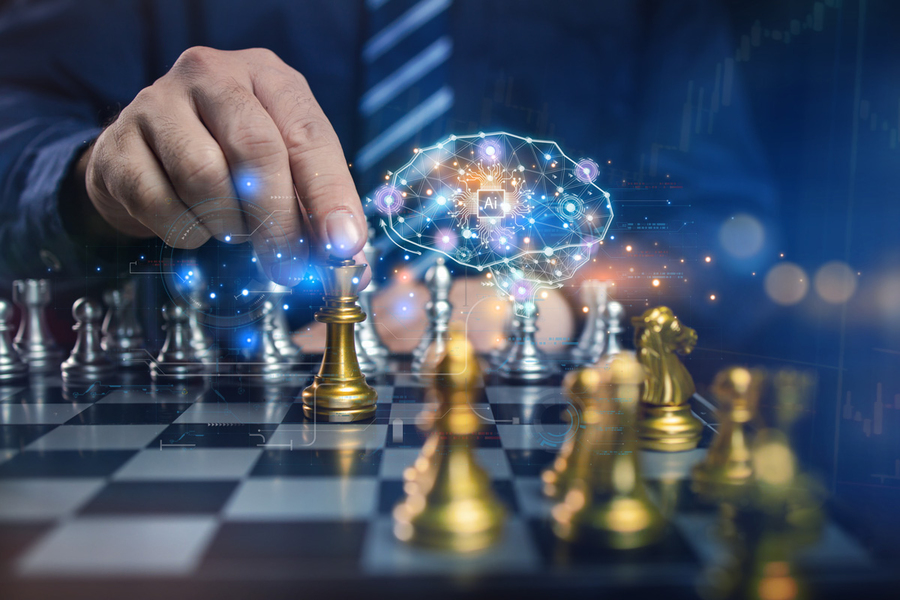
Challenges and Triumphs in AI-Predictive Accuracy
Key Findings from the Social Experiments
While the AI system showed promise in automating the generation and testing of social science theories, it faced challenges in directly predicting experimental outcomes. Initially, the AI tended to overestimate path estimates when asked to predict results without experimental data. However, when provided with data from actual experiments, the AI’s predictions improved significantly, highlighting the potential for enhanced accuracy through iterative learning and adjustment.
The Future of Social Science Research with AI Technologies
Improving Predictive Accuracy with Structural Insights
The study suggests that large language models contain latent information about human behaviour that can be effectively harnessed using structural causal models and simulation. This blend of AI and traditional research methods opens new avenues for understanding and predicting human behaviour in unprecedented ways, potentially revolutionizing how social science research is conducted.
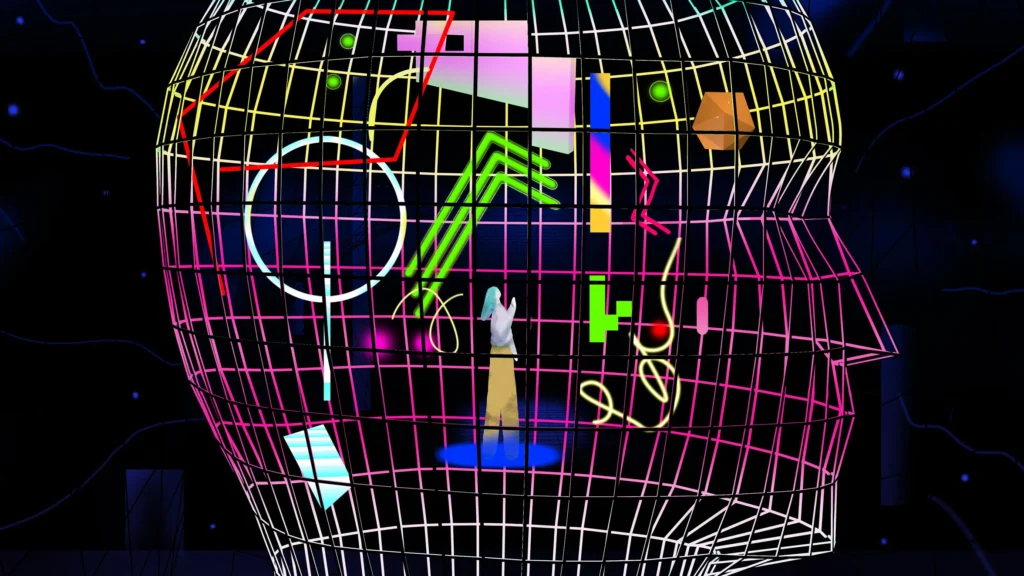
As MIT and Harvard continue to develop and refine this AI-powered system, the possibilities for future research are boundless. The integration of AI into social science research not only enhances the accuracy and efficiency of data analysis but also offers a new lens through which to view complex social dynamics. This technology heralds a new era of research, where machine learning and human insight combine to unlock deeper understandings of society and human behaviour.
For more detailed insights into this pioneering study and its implications for the future of social sciences, readers are encouraged to consult the full research paper linked here.